![]() 1st draft of python iterator Added Cifar10 and Cifar100 pybind port Change pybind to use IR for Skip and Manifest Signed-off-by: alex-yuyue <yue.yu1@huawei.com> DatasetNode as a base for all IR nodes namespace change Fix the namespace issue and make ut tests work Signed-off-by: alex-yuyue <yue.yu1@huawei.com> Add VOCDataset !63 Added RandomDataset * Added RandomDataset add imagefolder ir Pybind switch: CelebA and UT !61 CLUE example with class definition * Merge branch 'python-api' of gitee.com:ezphlow/mindspore into clue_class_pybind * Passing testcases * Added CLUE, not working add ManifestDataset IR Signed-off-by: alex-yuyue <yue.yu1@huawei.com> Update Coco & VOC & TFReader, Update clang-format, Reorder datasets_binding !69 Add Generator and move c_dataset.Iterator to dataset.Iterator * Add GeneratorDataset to c_dataset * Add GeneratorDataset to c_dataset !67 Moving c_datasets and adding sampler wrapper * Need to add create() method in datasets.py * migration from c_dataset to dataset part 1 !71 Fix indent error * Fix indentation error !72 Fix c_api tests cases * Fix c_api tests cases !73 Added CSV Dataset * Added CSVDataset pybind switch: Take and CelebA fixes !75 move c_dataset functionality to datasets * Fixed existing testcases * Added working clue and imagefolder * Added sampler conversion from pybind * Added sampler creation !77 Add Python API tree * Python API tree add minddataset TextFileDataset pybind Rename to skip test_concat.py and test_minddataset_exception.py !80 Add batch IR to python-api branch, most test cases work * staging III * staging, add pybind Enable more c_api take and CelebA tests; delete util_c_api !84 Schema changes in datasets.py * Schema changes !85 Remove input_indexes from sub-classes * remove input_index from each subclass !83 Remove C datasets * Removed c_dataset package * Remove c_datasets !82 pybind switch: shuffle * pybind switch: shuffle !86 Add build_vocab * Add build_vocab Rebase with upstream/master _shuffle conflict BatchNode error !88 Fix rebase problem * fix rebase problem Enable more unit tests; code typo/nit fixes !91 Fix python vocag hang * Fix python vocab hang !89 Added BucketBatchByLength Pybind switch * Added BucketBatchByLength Update and enable more tet_c_api_*.py tests !95 Add BuildSentencePeiceVocab * - Add BuildSentencePeiceVocab !96 Fix more tests * - Fix some tests - Enable more test_c_api_* - Add syncwait !99 pybind switch for device op * pybind switch for device op !93 Add getters to python API * Add getters to python API !101 Validate tree, error if graph * - Add sync wait !103 TFrecord/Random Datasets schema problem * - TfRecord/Random schem aproblem !102 Added filter pybind switch * Added Filter pybind switch !104 Fix num_samples * - TfRecord/Random schem aproblem !105 Fix to_device hang * Fix to_device hang !94 Adds Cache support for CLUE dataset * Added cache for all dataset ops * format change * Added CLUE cache support * Added Cache conversion Add save pybind fix compile err init modify concat_node !107 Fix some tests cases * Fix tests cases Enable and fix more tests !109 pybind switch for get dataset size * pybind_get_dataset_size some check-code fixes for pylint, cpplint and clang-format !113 Add callback * revert * dataset_sz 1 line * fix typo * get callback to work !114 Make Android compile clean * Make Android Compile Clean Fix build issues due to rebase !115 Fix more tests * Fix tests cases * !93 Add getters to python API fix test_profiling.py !116 fix get dataset size * fix get dataset size !117 GetColumnNames pybind switch * Added GetColumnNames pybind switch code-check fixes: clangformat, cppcheck, cpplint, pylint Delete duplicate test_c_api_*.py files; more lint fixes !121 Fix cpp tests * Remove extra call to getNext in cpp tests !122 Fix Schema with Generator * Fix Schema with Generator fix some cases of csv & mindrecord !124 fix tfrecord get_dataset_size and add some UTs * fix tfrecord get dataset size and add some ut for get_dataset_size !125 getter separation * Getter separation !126 Fix sampler.GetNumSamples * Fix sampler.GetNumSampler !127 Assign runtime getter to each get function * Assign runtime getter to each get function Fix compile issues !128 Match master code * Match master code !129 Cleanup DeviceOp/save code * Cleanup ToDevice/Save code !130 Add cache fix * Added cache fix for map and image folder !132 Fix testing team issues * Pass queue_name from python to C++ * Add Schema.from_json !131 Fix Cache op issues and delete de_pipeline * Roll back C++ change * Removed de_pipeline and passing all cache tests. * fixed cache tests !134 Cleanup datasets.py part1 * Cleanup dataset.py part1 !133 Updated validation for SentencePieceVocab.from_dataset * Added type_check for column names in SentencePieceVocab.from_dataset Rebase on master 181120 10:20 fix profiling temporary solution of catching stauts from Node.Build() !141 ToDevice Termination * ToDevice termination pylint fixes !137 Fix test team issues and add some corresponding tests * Fix test team issues and add some corresponding tests !138 TreeGetter changes to use OptPass * Getter changes to use OptPass (Zirui) Rebase fix !143 Fix cpplint issue * Fix cpplint issue pylint fixes in updated testcases !145 Reset exceptions testcase * reset exception test to master !146 Fix Check_Pylint Error * Fix Check_Pylint Error !147 fix android * fix android !148 ToDevice changes * Add ToDevice to the iterator List for cleanup at exit !149 Pylint issue * Add ToDevice to the iterator List for cleanup at exit !150 Pylint 2 * Add ToDevice to the iterator List for cleanup at exit !152 ExecutionTree error * ET destructor error !153 in getter_pass, only remove callback, without deleting map op * getter pass no longer removes map !156 early __del__ of iterator/to_device * early __del__ of iterator !155 Address review comments Eric 1 * Added one liner fix to validators.py * roll back signature fix * lint fix * Eric Address comments 2 * C++ lint fix * Address comments Eric 1 !158 Review rework for dataset bindings - part 1 * Reorder nodes repeat and rename * Review rework for dataset bindings - part 1 !154 Fixing minor problems in the comments (datasets.py, python_tree_consumer.cc, iterators_bindings.cc, and iterators.py) * Fixing minor problems in the comments (datasets.py, python_tree_consum… !157 add replace none * Add replace_none to datasets.py, address comments in tests Trying to resolve copy Override the deepcopy method of deviceop Create_ir_tree method Create_ir_tree method 2 Create_ir_tree method 2 del to_device if already exists del to_device if already exists cache getters shapes and types Added yolov3 relaxation, to be rolled back Get shapes and types together bypass yolo NumWorkers for MapOp revert Yolo revert Thor Print more info Debug code: Update LOG INFO to LOG ERROR do not remove epochctrl for getter pass Remove repeat(1) pritn batch size add log to tree_consumer and device_queue op Revert PR 8744 Signed-off-by: alex-yuyue <yue.yu1@huawei.com> __del__ toDEvice __del__ toDevice2 !165 add ifndef ENABLE_ANDROID to device queue print * Add ifndef ENABLE_ANDROID to device queue print revert some changes !166 getter: get_data_info * getter: get_data_info !168 add back tree print * revert info to warnning in one log * add back the missed print tree log Release GIL in GetDataInfo |
||
---|---|---|
.gitee | ||
.github | ||
akg@6ffe9c2431 | ||
cmake | ||
config | ||
docker | ||
docs | ||
graphengine@383f7f751d | ||
include | ||
mindspore | ||
model_zoo | ||
scripts | ||
serving | ||
tests | ||
third_party | ||
.clang-format | ||
.gitignore | ||
.gitmodules | ||
CMakeLists.txt | ||
CONTRIBUTING.md | ||
LICENSE | ||
NOTICE | ||
README.md | ||
README_CN.md | ||
RELEASE.md | ||
SECURITY.md | ||
Third_Party_Open_Source_Software_Notice | ||
build.bat | ||
build.sh | ||
requirements.txt | ||
setup.py |
README.md
What Is MindSpore
MindSpore is a new open source deep learning training/inference framework that could be used for mobile, edge and cloud scenarios. MindSpore is designed to provide development experience with friendly design and efficient execution for the data scientists and algorithmic engineers, native support for Ascend AI processor, and software hardware co-optimization. At the meantime MindSpore as a global AI open source community, aims to further advance the development and enrichment of the AI software/hardware application ecosystem.

For more details please check out our Architecture Guide.
Automatic Differentiation
There are currently three automatic differentiation techniques in mainstream deep learning frameworks:
- Conversion based on static compute graph: Convert the network into a static data flow graph at compile time, then turn the chain rule into a data flow graph to implement automatic differentiation.
- Conversion based on dynamic compute graph: Record the operation trajectory of the network during forward execution in an operator overloaded manner, then apply the chain rule to the dynamically generated data flow graph to implement automatic differentiation.
- Conversion based on source code: This technology is evolving from the functional programming framework and performs automatic differential transformation on the intermediate expression (the expression form of the program during the compilation process) in the form of just-in-time compilation (JIT), supporting complex control flow scenarios, higher-order functions and closures.
TensorFlow adopted static calculation diagrams in the early days, whereas PyTorch used dynamic calculation diagrams. Static maps can utilize static compilation technology to optimize network performance, however, building a network or debugging it is very complicated. The use of dynamic graphics is very convenient, but it is difficult to achieve extreme optimization in performance.
But MindSpore finds another way, automatic differentiation based on source code conversion. On the one hand, it supports automatic differentiation of automatic control flow, so it is quite convenient to build models like PyTorch. On the other hand, MindSpore can perform static compilation optimization on neural networks to achieve great performance.

The implementation of MindSpore automatic differentiation can be understood as the symbolic differentiation of the program itself. Because MindSpore IR is a functional intermediate expression, it has an intuitive correspondence with the composite function in basic algebra. The derivation formula of the composite function composed of arbitrary basic functions can be derived. Each primitive operation in MindSpore IR can correspond to the basic functions in basic algebra, which can build more complex flow control.
Automatic Parallel
The goal of MindSpore automatic parallel is to build a training method that combines data parallelism, model parallelism, and hybrid parallelism. It can automatically select a least cost model splitting strategy to achieve automatic distributed parallel training.
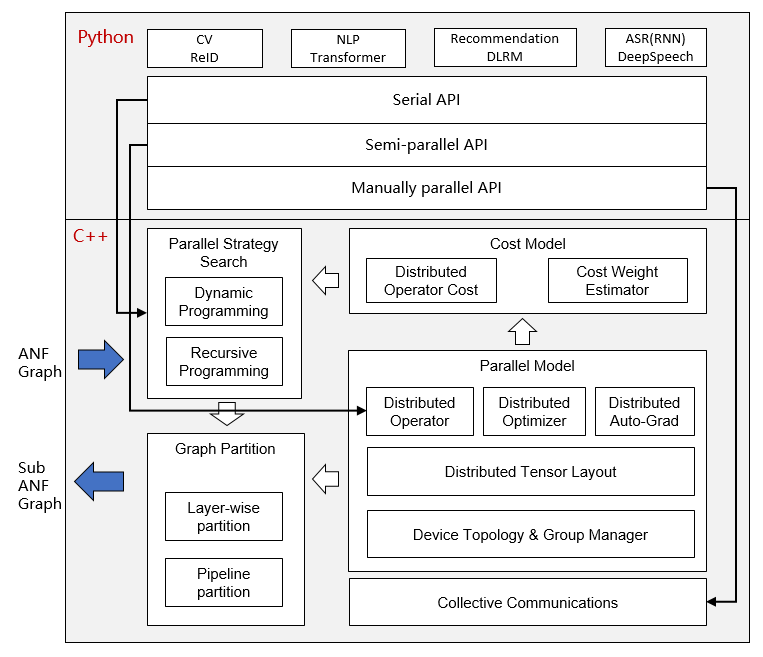
At present, MindSpore uses a fine-grained parallel strategy of splitting operators, that is, each operator in the figure is splitted into a cluster to complete parallel operations. The splitting strategy during this period may be very complicated, but as a developer advocating Pythonic, you don't need to care about the underlying implementation, as long as the top-level API compute is efficient.
Installation
Binaries
MindSpore offers build options across multiple backends:
Hardware Platform | Operating System | Status |
---|---|---|
Ascend910 | Ubuntu-x86 | ✔️ |
Ubuntu-aarch64 | ✔️ | |
EulerOS-x86 | ✔️ | |
EulerOS-aarch64 | ✔️ | |
CentOS-x86 | ✔️ | |
CentOS-aarch64 | ✔️ | |
GPU CUDA 10.1 | Ubuntu-x86 | ✔️ |
CPU | Ubuntu-x86 | ✔️ |
Ubuntu-aarch64 | ✔️ | |
Windows-x86 | ✔️ |
For installation using pip
, take CPU
and Ubuntu-x86
build version as an example:
-
Download whl from MindSpore download page, and install the package.
pip install https://ms-release.obs.cn-north-4.myhuaweicloud.com/1.0.0/MindSpore/cpu/ubuntu_x86/mindspore-1.0.0-cp37-cp37m-linux_x86_64.whl
-
Run the following command to verify the install.
import numpy as np import mindspore.context as context import mindspore.nn as nn from mindspore import Tensor from mindspore.ops import operations as P context.set_context(mode=context.GRAPH_MODE, device_target="CPU") class Mul(nn.Cell): def __init__(self): super(Mul, self).__init__() self.mul = P.Mul() def construct(self, x, y): return self.mul(x, y) x = Tensor(np.array([1.0, 2.0, 3.0]).astype(np.float32)) y = Tensor(np.array([4.0, 5.0, 6.0]).astype(np.float32)) mul = Mul() print(mul(x, y))
[ 4. 10. 18.]
From Source
Docker Image
MindSpore docker image is hosted on Docker Hub, currently the containerized build options are supported as follows:
Hardware Platform | Docker Image Repository | Tag | Description |
---|---|---|---|
CPU | mindspore/mindspore-cpu |
x.y.z |
Production environment with pre-installed MindSpore x.y.z CPU release. |
devel |
Development environment provided to build MindSpore (with CPU backend) from the source, refer to https://www.mindspore.cn/install/en for installation details. |
||
runtime |
Runtime environment provided to install MindSpore binary package with CPU backend. |
||
GPU | mindspore/mindspore-gpu |
x.y.z |
Production environment with pre-installed MindSpore x.y.z GPU release. |
devel |
Development environment provided to build MindSpore (with GPU CUDA10.1 backend) from the source, refer to https://www.mindspore.cn/install/en for installation details. |
||
runtime |
Runtime environment provided to install MindSpore binary package with GPU CUDA10.1 backend. |
||
Ascend | — | — | Coming soon. |
NOTICE: For GPU
devel
docker image, it's NOT suggested to directly install the whl package after building from the source, instead we strongly RECOMMEND you transfer and install the whl package inside GPUruntime
docker image.
-
CPU
For
CPU
backend, you can directly pull and run the latest stable image using the below command:docker pull mindspore/mindspore-cpu:1.0.0 docker run -it mindspore/mindspore-cpu:1.0.0 /bin/bash
-
GPU
For
GPU
backend, please make sure thenvidia-container-toolkit
has been installed in advance, here are some install guidelines forUbuntu
users:DISTRIBUTION=$(. /etc/os-release; echo $ID$VERSION_ID) curl -s -L https://nvidia.github.io/nvidia-docker/gpgkey | apt-key add - curl -s -L https://nvidia.github.io/nvidia-docker/$DISTRIBUTION/nvidia-docker.list | tee /etc/apt/sources.list.d/nvidia-docker.list sudo apt-get update && sudo apt-get install -y nvidia-container-toolkit nvidia-docker2 sudo systemctl restart docker
Then edit the file daemon.json:
$ vim /etc/docker/daemon.json { "runtimes": { "nvidia": { "path": "nvidia-container-runtime", "runtimeArgs": [] } } }
Restart docker again:
sudo systemctl daemon-reload sudo systemctl restart docker
Then you can pull and run the latest stable image using the below command:
docker pull mindspore/mindspore-gpu:1.0.0 docker run -it -v /dev/shm:/dev/shm --runtime=nvidia --privileged=true mindspore/mindspore-gpu:1.0.0 /bin/bash
To test if the docker image works, please execute the python code below and check the output:
import numpy as np import mindspore.context as context from mindspore import Tensor from mindspore.ops import functional as F context.set_context(mode=context.PYNATIVE_MODE, device_target="GPU") x = Tensor(np.ones([1,3,3,4]).astype(np.float32)) y = Tensor(np.ones([1,3,3,4]).astype(np.float32)) print(F.tensor_add(x, y))
[[[ 2. 2. 2. 2.], [ 2. 2. 2. 2.], [ 2. 2. 2. 2.]], [[ 2. 2. 2. 2.], [ 2. 2. 2. 2.], [ 2. 2. 2. 2.]], [[ 2. 2. 2. 2.], [ 2. 2. 2. 2.], [ 2. 2. 2. 2.]]]
If you want to learn more about the building process of MindSpore docker images, please check out docker repo for the details.
Quickstart
See the Quick Start to implement the image classification.
Docs
More details about installation guide, tutorials and APIs, please see the User Documentation.
Community
Governance
Check out how MindSpore Open Governance works.
Communication
- MindSpore Slack - Communication platform for developers.
- IRC channel at
#mindspore
(only for meeting minutes logging purpose) - Video Conferencing: TBD
- Mailing-list: https://mailweb.mindspore.cn/postorius/lists
Contributing
Welcome contributions. See our Contributor Wiki for more details.
Maintenance phases
Project stable branches will be in one of the following states:
State | Time frame | Summary |
---|---|---|
Planning | 1 - 3 months | Features are under planning. |
Development | 3 months | Features are under development. |
Maintained | 6 - 12 months | All bugfixes are appropriate. Releases produced. |
Unmaintained | 0 - 3 months | All bugfixes are appropriate. No Maintainers and No Releases produced. |
End Of Life (EOL) | N/A | Branch no longer accepting changes. |
Maintenance status
Branch | Status | Initial Release Date | Next Phase | EOL Date |
---|---|---|---|---|
r1.1 | Development | 2020-12-31 estimated | Maintained 2020-12-31 estimated |
|
r1.0 | Maintained | 2020-09-24 | Unmaintained 2021-03-30 estimated |
|
r0.7 | Maintained | 2020-08-31 | Unmaintained 2020-11-30 estimated |
|
r0.6 | Unmaintained | 2020-07-31 | End Of Life 2020-12-30 estimated |
|
r0.5 | Maintained | 2020-06-30 | Unmaintained 2021-06-30 estimated |
|
r0.3 | End Of Life | 2020-05-31 | 2020-09-30 | |
r0.2 | End Of Life | 2020-04-30 | 2020-08-31 | |
r0.1 | End Of Life | 2020-03-28 | 2020-06-30 |
Release Notes
The release notes, see our RELEASE.